
A new light on neural connections
May 7, 2025
Michał Januszewski, Research Scientist, and Viren Jain, Research Scientist
In collaboration with the Institute of Science and Technology Austria (ISTA), we published in Nature the first-ever method for using light microscopy to comprehensively map all the neurons and their connections in a block of brain tissue. The key finding from this validation experiment is that this approach works as well as electron microscopy-based connectomics.
Quick links
In the 1660s, with the help of a simple, homemade light microscope that magnified samples more than 250 times, a Dutch fabric merchant named Antoine van Leeuwenhoek became the first person to document a close-up view of bacteria, red blood cells, sperm cells, and many other scientific sights. Since then, light microscopy has solidified its place as a bedrock technique in our quest to understand living organisms. Today, it is nearly ubiquitous in life science laboratories, enabling biologists to identify and characterize cells, organs and tissues and to diagnose many diseases.
One field that light microscopy has not managed to penetrate, however, is connectomics — an area of neuroscience in which Google has made fundamental contributions over the past decade. Efforts to comprehensively map all the neurons in a region — including our previous connectomics work — have instead relied on a technique called electron microscopy, which can capture an extremely close-up view of structural information within a cell. Electron microscopy has a major limitation, however: it requires expensive, highly specialized equipment that is not readily accessible to most neuroscience labs.
Today, in collaboration with colleagues at the Institute of Science and Technology Austria (ISTA), we published in the journal Nature, “Light-microscopy based connectomic reconstruction of mammalian brain tissue”, in which we report the first-ever method for using light microscopy to comprehensively map all the neurons and their connections in a block of mouse brain tissue. We achieved this by customizing several well-established and validated techniques and combining them into a single workflow that we call LICONN (light microscopy-based connectomics). Our colleagues at ISTA led the project’s key innovation — a protocol that physically expands brain tissue while preserving structural integrity, and at the same time chemically labels all proteins in order to provide the image contrast necessary for tracing neurons and detecting other cellular structures such as synapses.
We iterated with ISTA on the details of the protocol, applying our suite of image analysis and machine learning (ML) tools for connectomics, and ultimately validating LICONN at scale by providing an automated reconstruction of a nearly one-million cubic micron volume of mouse cortex. We then comprehensively verified the traceability of all ~0.5 meters of neurites packed within a smaller volume of mouse hippocampus tissue, demonstrating that LICONN works comparably well to electron microscope–based connectomics. We also showed that LICONN unlocks the ability to simultaneously measure structural and molecular information in a tissue sample, which will enable fundamental new opportunities to understand the workings of the brain.

Connectomics utilizes microscopy-based imaging coupled with computational processing to reconstruct individual cells and their intricate connections within brain tissue.
The need for new approaches
Electron microscopes produce images based on how a beam of electrons scatters when it interacts with a sample, enabling it to have a much higher resolution than light microscopy — it can image features on the scale of a nanometer compared to hundreds of nanometers for (visible) light microscopy. But despite light microscopy’s limited resolution, its accessibility is a major advantage. The electron microscopes used for connectomics research can cost millions of dollars, and operating them requires extensive specialized training, making them often available only to neuroscientists at large, well-funded institutions.
Researchers have used a handful of “super-resolution” light microscopy techniques, which can image beyond light’s diffraction limit to achieve nanoscale resolution, to label and trace relatively small subsets of neurons in various ways. But these techniques have not been able to achieve “dense” connectomics — i.e., mapping all densely packed neurons at the scale of the finest fibers.
A key benefit of light microscopy is that it can capture light at many different wavelengths, so researchers can use it to visualize a rainbow of fluorescent markers for proteins, neurotransmitters, and other molecules that provide clues about how neurons and circuits function or malfunction. LICONN unlocks the ability to align these molecular markers with detailed structural maps of neuronal pathways with unprecedented precision and ease, which will help yield important insights into how the brain generates cognition, perception and behavior.
Expanding the possibilities
To get around the problem of limited resolution with light microscopy, our ISTA colleagues turned their efforts toward the sample itself. They used a technique called expansion microscopy, which uses a substance called a hydrogel (the same material that makes diapers absorbent). Hydrogels absorb moisture by crosslinking with water molecules, swelling as they do so — a principle that researchers at the Boyden Lab at the Massachusetts Institute of Technology relied on to develop a tissue expansion protocol for microscopy in 2015.
Although expansion microscopy is used in many labs today, existing tissue expansion protocols do not preserve the tissue well enough to trace densely labeled neuronal structures, so our ISTA collaborators developed a new expansion protocol for LICONN. It involved first cutting a tiny block of tissue into 50 micrometer sections, then treating each section with a sequence of three different hydrogels. Two of the hydrogels created distinct, interweaving polymer networks within the tissue, with each one expanding the tissue by a factor of four, and the third hydrogel served to stabilize those networks. With this protocol, the tissue expanded by about 16 times in each direction, which is loosely comparable to starting with a sugar cube and ending up with a box of tissues.
To make the neurons visible, our colleagues incubated the brain tissue sections with a green fluorescent dye, effectively "labeling" all proteins inside cells. In some samples, they also used several other dyes that home in on specific proteins, neurotransmitters or other molecules that are important in determining a neuron’s cell type or function.
Each tissue section is essentially a serial slice of a thick swathe of neurons enmeshed with other cells such as glia. Tracing this dense mass of brain cell fragments in expanded tissue can be challenging. We accomplished this using algorithms that we have previously developed for automated reconstruction of neurons through multiple tissue slices. These tools have achieved state-of-the-art accuracy for delineating distinct structures within brain tissue images acquired with electron microscopy (flood-filling networks) and for aligning and stitching together serial electron microscopy images (SOFIMA).
Validating LICONN
A chief aim of our study was to demonstrate that LICONN is as robust as electron microscopy–based connectomics. First, we showed that our results matched closely with manual tracing of all the sprawling dendrites and thin, twisty axons within a subsection of tissue. We then trained our automated reconstruction algorithm on the manually drawn data. After optimizing the algorithm’s accuracy, we showed that it performed comparably to reconstructions from electron microscopy data.
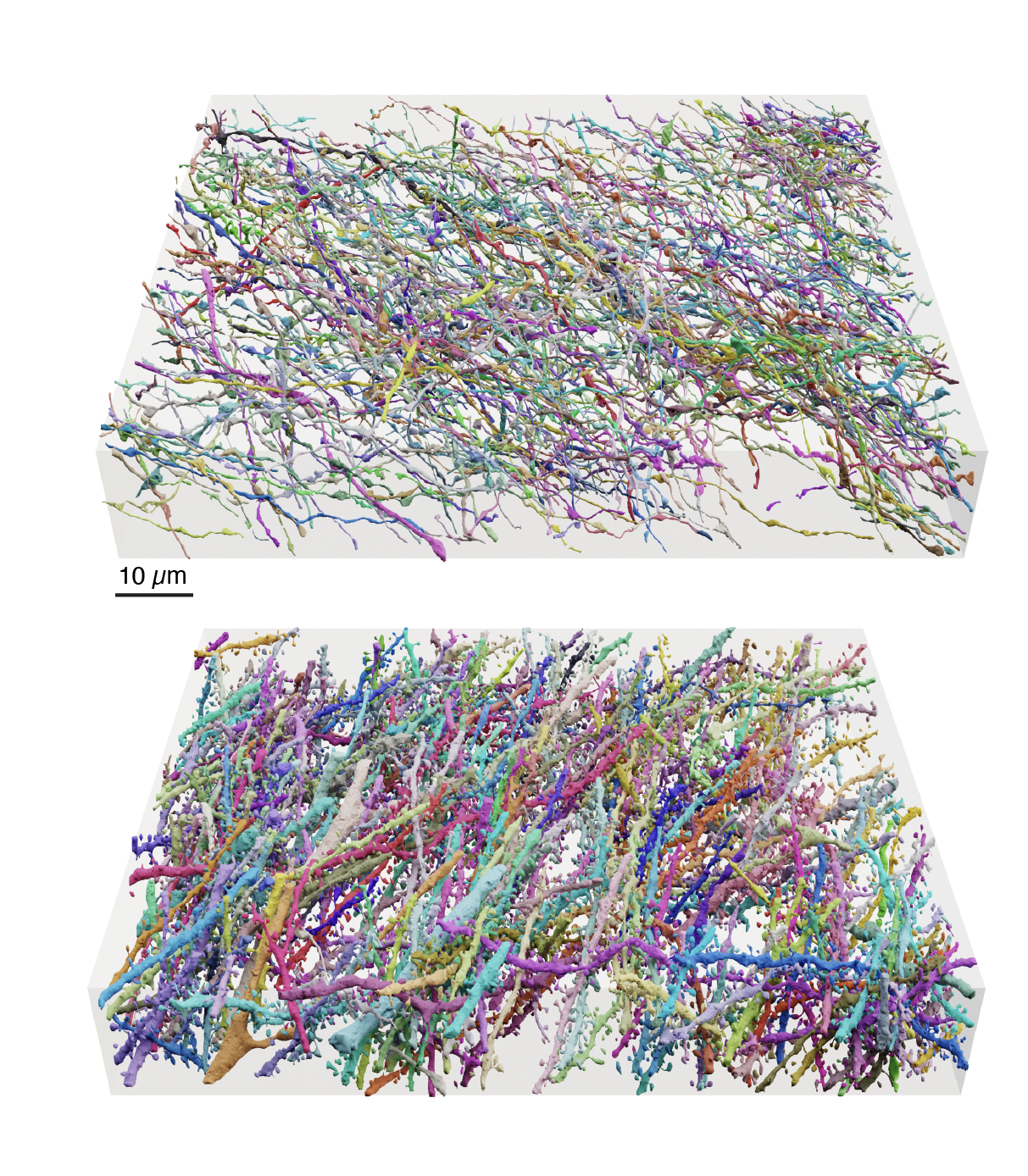
Renderings of the reconstructed LICONN volume with automated segmentation from flood-filling networks. The image depicts a reconstruction of 5.8% of axons (top) and 27.3% of dendrites and a small number of axons (bottom) from the original tissue volume.
Next, we confirmed that synaptic connections are robustly identifiable in LICONN volumes. LICONN’s multimodal capabilities — to simultaneously image structure (morphology), connectivity, and also specific molecules of interest within the same sample — enabled us to directly label specific proteins that distinguish pre-synaptic versus post-synaptic regions in the tissue. We were further able to use these labels as ground truth for training ML algorithms to identify these synaptic areas purely from structural observations, as is done in electron microscopy connectomics.
Finally, we also used protein labeling to reveal molecular information in connectomics maps that cannot be reliably gleaned from structure alone, thus going well beyond the capabilities of electron microscopy connectomics. For example, labeling proteins associated with two different neurotransmitters allowed us to directly differentiate between inhibitory and excitatory synapses. Labeling yet another protein pinpointed electrical synapses, which are ubiquitous in the nervous system, but are typically neglected from electron microscopy–based connectomic reconstructions due to their small and subtle structural appearance. The ability to conclusively identify these molecular features in brain tissue, and place them in the context of overall brain morphology and connectivity, will significantly expand the utility of connectomic maps.

3D-rendering and magnified views of a dendrite (segmented with flood filling networks) and excitatory synaptic connections (white bars) detected via immunolabeling.
In our study, the block of mouse brain we mapped was just a small percentage of the 1 square millimeter block of human brain tissue, about the size of a grain of rice, that we mapped with colleagues at Harvard University last year using electron microscopy–based connectomics. We are currently working on scaling up LICONN to be able to capture data from larger tissue volumes.
The path ahead
We recently announced a joint effort to map a mouse brain, starting with the hippocampus — one of several connectomics collaborations funded by the National Institutes of Health. We are also working towards understanding how brain structures change in the context of diseases like Alzheimer's. However, fully achieving these goals will require innovations that make brain mapping more efficient by orders of magnitude.
The validation of LICONN takes a clear step toward that goal, holding great promise both to increase the accessibility of connectomics and to enable scientists to extract “multimodal” connectomes that combine information about neurons and synapses with molecular details that have previously been inaccessible. LICONN’s utility to the neuroscience community is evidenced by the fact that already, several labs have successfully replicated the technique based on our preprint, first posted in March 2024. We see this work as a step toward achieving high-level goals of neuroscience that could unlock important applications in the clinic and beyond.
Acknowledgements
We thank our academic collaborators in the Danzl Lab (ISTA), and acknowledge core contributions from the Connectomics Team at Google. We are grateful to Alla Katsnelson and Elise Kleeman for their help. Thanks to Lizzie Dorfman, Michael Brenner, John Platt, and Yossi Matias for their support, coordination and leadership.
-
Labels:
- General Science
- Health & Bioscience